Data-Driven Maintenance

Why the current approach falls short
These traditional methods of maintenance are becoming increasingly misaligned with the contemporary needs and challenges of asset management. This results in two significant pitfalls:
Missed Issues
Misaligned objectives
A new era of maintenance
Data-Driven Maintenance (DDM) is the integration of advanced analytics into the realm of property maintenance. Unlike traditional strategies, which rely heavily on preset schedules or reactionary measures, DDM employs continuous data collection and analysis to guide maintenance decisions. DA19 summarises the workflow of this smarter form of maintenance as: “analytics pulled from big data, relayed by wireless sensor networks, analysed by cloud-based algorithms and visualised on mobile computing devices” which is effectively “changing maintenance, disrupting traditional practices and creating new value opportunities.”
DDM is grounded in the principle of continuously monitoring the billions of data points produced by operational plant and equipment. Real-time in nature, as data is gathered, it's simultaneously normalised and analysed. This ongoing monitoring allows for an ever-updated operational snapshot of a building, allowing for issues or dips in performance to be flagged as soon as they arise.
At the heart of DDM lies sophisticated data analysis. This isn't just about collecting numbers but about understanding them. Advanced algorithm-based rules process this continuous stream of data, deciphering patterns, spotting anomalies, and making sense of the vast amount of information flowing in.
Instead of a broad preventive stance, where checks are made based on set intervals, DDM utilises the analysed data to predict when and where maintenance might be needed next. It's a shift from 'time-based' to 'condition-based' checks. Further, While DDM is largely proactive, it doesn't discard reactive measures. Instead, these reactive actions are informed by data-backed alerts rather than tenant complaints or anecdotal feedback.
DDM is inherently adaptive. As data flows in and is analysed, the derived insights might prompt changes to maintenance schedules or resource allocations. This adaptability ensures that the maintenance approach is always in tune with the building's current needs and critical issues are prioritised based on impact.
It's crucial to note that DDM isn't just about data. It's also about the technological ecosystem that facilitates this data-driven approach. From the sensors that gather data to the cloud infrastructures that store it, and the analytics software that analyse it – each plays a pivotal role in bringing DDM to life.
Phases of implementation

1. Initial integration
Initial integration of analytics and optimisation tools, which may add to initial expenses but set the foundation for future savings.

2. Contract evolution
The previously separate costs for analytics and optimisation now become part of the core contract, with the added benefit of reduced routine maintenance expenses.

3. Mastery and refinement
The system operates at peak efficiency, leading to further reductions in costs, heightened equipment lifespan, and increased tenant satisfaction.
The benefits of data-driven maintenance
Optimised maintenance contracts
Reduced equipment downtime
Extension of equipment life cycle
Improved sustainability
Contractor accountability
Team productivity
In our client’s own words
Powering property teams in these world leading companies.

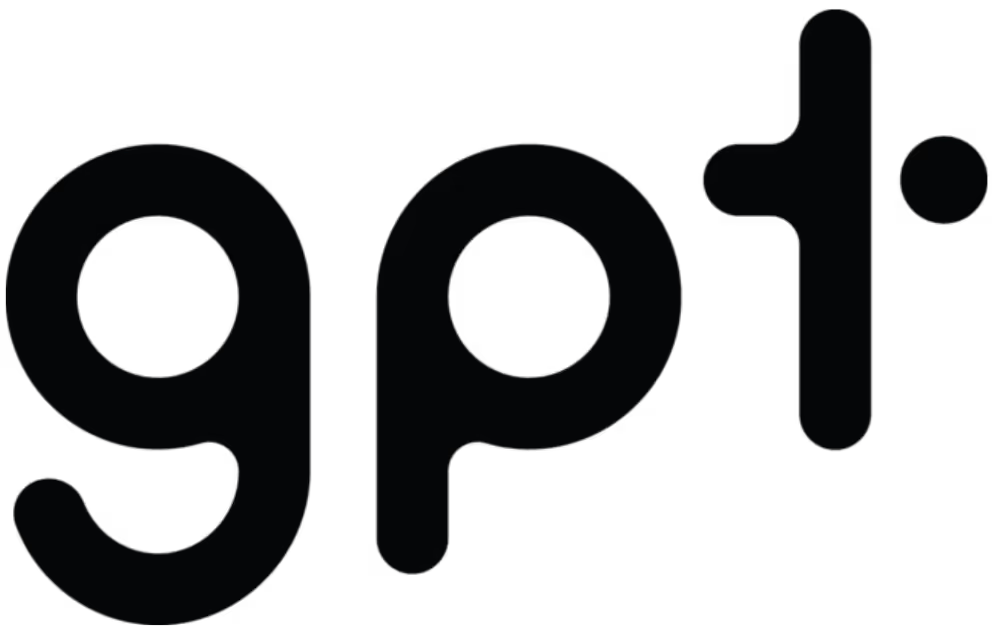
